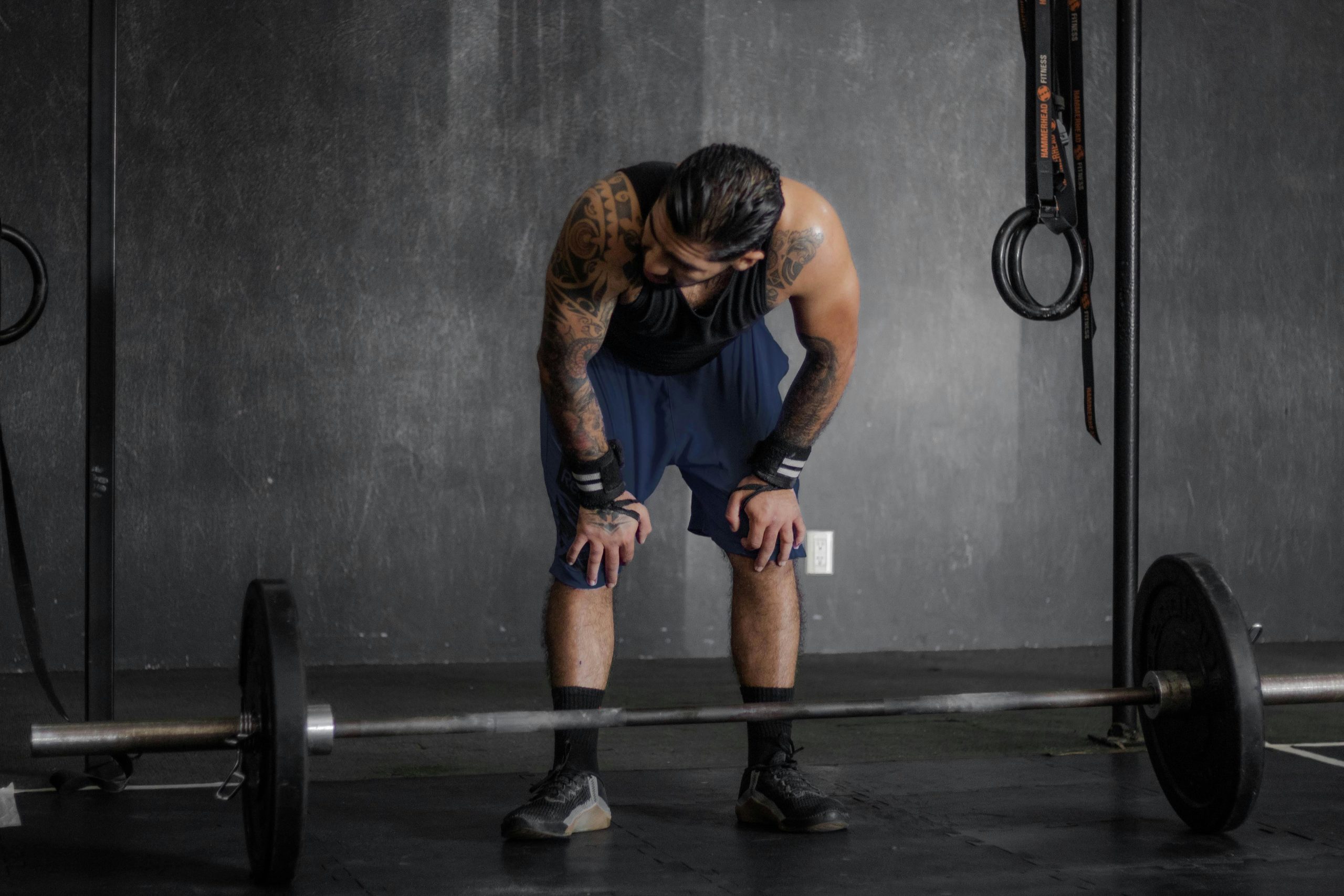
In the digital age, social media has become a powerful tool for brands, influencers, and individuals alike, providing a platform to express opinions, share experiences, and engage with audiences. However, with the vast amount of content generated daily, understanding public sentiment accurately has become a formidable challenge. This blog post delves into the complexities of measuring social media sentiment, exploring key challenges such as detecting sarcasm, adapting to evolving slang, and the need for domain-specific models. We will also discuss how tools like Sociobo can help brands navigate these challenges effectively.
Understanding Social Media Sentiment Analysis
Social media sentiment analysis refers to the process of using natural language processing (NLP) and machine learning techniques to analyze online conversations and gauge public opinion about a brand, product, or topic. By interpreting the emotions and sentiments expressed in social media posts, businesses can gain valuable insights into customer feelings and brand perception.
Despite its potential, sentiment analysis faces significant hurdles that can lead to misinterpretations and inaccuracies. Let’s explore some of these challenges in detail.
The Challenge of Detecting Sarcasm and Irony
One of the most significant challenges in sentiment analysis is accurately detecting sarcasm and irony. These forms of expression are prevalent on social media, where users often employ humor or exaggeration to convey their feelings. Unfortunately, traditional sentiment analysis models often struggle to interpret these nuanced statements, leading to misclassification of sentiments.
The Complexity of Sarcasm
Sarcasm can drastically alter the intended meaning of a statement. For instance, a tweet that reads, “Oh great, another rainy day,” could be interpreted as a positive sentiment by an automated system, whereas the user may actually be expressing frustration. This misinterpretation can skew overall analysis results, leading brands to make misguided decisions based on inaccurate data.
Advanced Techniques for Sarcasm Detection
Researchers have developed specialized techniques to improve sarcasm detection in sentiment analysis. One approach involves ensemble classification methods, which combine multiple machine learning algorithms to analyze linguistic features indicative of sarcasm. For example, studies have employed Random Forest, K-Nearest Neighbor, and Naive Bayes classifiers to achieve improved accuracy in identifying sarcastic statements.
Another promising strategy is the use of deep multi-task learning frameworks. By simultaneously training models for both sentiment analysis and sarcasm detection, these frameworks leverage the correlation between the two tasks, resulting in enhanced performance. Some studies have reported up to a 94% accuracy rate in sentiment classification using these advanced methods.
Key Features for Sarcasm Detection
To enhance sarcasm detection, advanced systems incorporate several key features:
- Contextual Analysis: Examining surrounding text to identify inconsistencies or contradictions that may indicate sarcasm.
- Lexical Cues: Identifying specific words or phrases commonly associated with sarcastic expressions.
- Punctuation and Capitalization Patterns: Recognizing unusual punctuation or capitalization that may signal sarcasm.
- Sentiment Contrast: Detecting conflicts between the literal sentiment of words and the overall intended meaning.
Despite these advancements, the challenge of detecting sarcasm and irony remains significant. The complexity of human language, cultural nuances, and the ever-evolving nature of online communication continue to pose difficulties for even the most sophisticated algorithms.
Adapting to Evolving Slang
Another critical challenge in social media sentiment analysis is adapting to the rapid evolution of slang. Social media platforms, particularly those popular among younger users like TikTok, are breeding grounds for new expressions and linguistic innovations. As language evolves, traditional lexicons and models can quickly become obsolete.
The Need for Dynamic Lexicons
To address this challenge, sentiment analysis systems are increasingly incorporating dynamic lexicons that are continuously updated to include new slang terms and expressions. These lexicons can be crowd-sourced or automatically generated from large-scale social media data, ensuring that sentiment analysis tools remain relevant and accurate.
Machine Learning Adaptation
Advanced machine learning models are being trained on continuously updated datasets, allowing them to learn and adapt to new slang terms and their associated sentiments in real-time. This approach helps maintain the relevance and accuracy of sentiment analysis tools as the language continues to evolve.
Context-Aware Algorithms
Sophisticated algorithms are being developed to understand the context in which new slang terms are used. By analyzing surrounding words, sentence structure, and even multimedia elements like emojis and hashtags, these algorithms can infer the sentiment behind novel expressions.
Platform-Specific Models
Recognizing that slang can vary significantly across different social media platforms, researchers are developing platform-specific sentiment analysis models. For example, models tailored for TikTok might focus on audio-visual cues and short-form content, while Twitter-specific models might emphasize hashtag usage and thread context.
User Feedback Integration
Many sentiment analysis tools now incorporate user feedback mechanisms, allowing for rapid correction and learning when new slang terms are misinterpreted. This human-in-the-loop approach helps bridge the gap between automated systems and the ever-changing landscape of social media language.
Despite these advancements, the challenge of adapting to evolving slang remains significant. The informal and creative nature of social media communication means that new terms and expressions can emerge and spread rapidly.
Domain-Specific Sentiment Models
As businesses increasingly seek to leverage sentiment analysis for industry-specific insights, domain-specific sentiment models have emerged as a crucial solution. These models are tailored to understand the unique vocabulary, jargon, and context-specific sentiment patterns within particular industries.
Financial Sentiment Analysis
In the financial sector, specialized models like Bloomberg-GPT and FinBERT have been developed to tackle the complexities of financial sentiment analysis. These models are trained on vast amounts of financial news and data points, demonstrating superior performance compared to general-purpose language models in financial sentiment tasks.
Healthcare and Technology Applications
Domain-specific sentiment models are also being developed in healthcare and technology. In healthcare, models are trained on medical literature, patient reviews, and clinical notes to better understand health-related sentiments. Similarly, in the technology sector, models are fine-tuned to interpret sentiment in technical discussions, product reviews, and developer forums.
Strategies for Creating Effective Domain-Specific Models
To create effective domain-specific models, researchers employ several strategies:
- Specialized Training Data: Models are trained on large datasets specific to the domain, including industry reports and specialized social media content.
- Custom Sentiment Lexicons: Domain-specific sentiment lexicons are developed to capture the unique terminology and sentiment associations within the field.
- Fine-Tuning Pre-Trained Models: General-purpose language models are fine-tuned on domain-specific data to adapt their understanding to the particular field.
- Incorporating Domain Knowledge: Expert knowledge is integrated into the models to improve their understanding of domain-specific contexts and nuances.
- Addressing Domain-Specific Challenges: Models are designed to handle challenges unique to the domain, such as interpreting financial metrics or medical terminology.
The use of domain-specific sentiment models has led to significant improvements in sentiment analysis accuracy within specialized fields. For instance, in financial sentiment analysis, these models can better interpret complex financial statements, earnings reports, and market commentary, providing more reliable insights for investors and analysts.
The Role of Sociobo in Sentiment Analysis
As brands navigate the complexities of social media sentiment analysis, tools like Sociobo can be invaluable. Sociobo is designed to help individuals and businesses leverage social media to build their personal or brand identity effectively. By employing a technique known as “social proof aggregation,” Sociobo enhances a brand’s visibility, authority, and influence on social media platforms.
Leveraging Social Proof
Sociobo’s approach to social proof is rooted in the psychological phenomenon identified by Dr. Robert Cialdini, where individuals look to the actions and beliefs of others when uncertain about what to do. In social media, high engagement metrics, such as followers, likes, and shares, suggest credibility and popularity, attracting more genuine engagement.
By providing aggregated followers—high-quality bot accounts created using official apps—Sociobo boosts engagement rates and improves profile discovery. This positive impact on trust scores in social media algorithms can help brands attract real followers and enhance their overall social media performance.
Customized Experience Packages
Sociobo offers customized experience packages for various platforms, including Instagram, TikTok, YouTube, Facebook, and Spotify. By tailoring services to meet the unique needs of each platform, Sociobo enables brands to navigate the challenges of social media sentiment analysis more effectively.
Enhancing Brand Presence
The services offered by Sociobo are particularly beneficial for individuals and brands looking to establish or grow their presence on social media. By leveraging social proof, brands can enhance their credibility and influence, making them more attractive to organic followers.
Measuring social media sentiment is a complex yet essential task for brands and individuals seeking to understand public opinion and enhance their online presence. While challenges such as detecting sarcasm, adapting to evolving slang, and developing domain-specific models persist, advancements in technology and methodologies offer promising solutions.
As you navigate the intricate landscape of social media sentiment analysis, consider leveraging tools like Sociobo to enhance your brand’s visibility and authority. By employing social proof aggregation, you can attract genuine engagement and build a strong online presence that resonates with your audience.
If you’re ready to elevate your social media strategy and overcome the challenges of sentiment analysis, explore Sociobo’s services today at Sociobo.com. Your brand deserves to shine in the crowded digital landscape!
No comment yet, add your voice below!